With the continued exponential growth of online shopping, retailers know how important a search engine is to drive sales on their e-commerce site. Yet, did you know that search bar shoppers are 2-3x more likely to make a purchase than shoppers who do not use it? Whether your search engine is Bloomreach, Algolia, Salesforce or SearchSpring, just to name a few, there are a number of ways you can get even more out of your search engine and provide the most relevant search results with Lily AI, leading your customers to convert at a higher rate.
Synonyms and Semantic Search
More often than not, search engines don’t provide a shopper-friendly search experience, because they insist that users search for products using the precise product name. In reality, there’s a big difference in how the product information like product titles and descriptions are curated vs how shoppers actually search for them in the real world.
Every shopper will search uniquely for an item. While I might be searching for the perfect ‘oversized sweater’ for fall, you might be searching for a ‘loose sweater’ or ‘oversized pullover’, or ‘slouchy sweater’ when shopping on your favorite e-commerce site. We all have the same intent of wanting to purchase a sweater for the fall, but we have each described it in different language that is unique to us.
An e-commerce site search engine on its own will typically not offer semantic search; or, if they do, it will be very basic synonym mapping. This is because a great deal of domain expertise and the inclusion of trending keywords/search-terms needs to go into finding accurate synonyms, particularly for the fashion, beauty and home industries. With Lily AI enriched product attribution data directly linked into an e-commerce site search engine, your high intent shoppers who are using the search bar are going to see much more relevant search results with the synonym mapping we provide and the domain expertise that goes into each and every attribute in our taxonomy. (Learn here how this led to an increase in product discovery by 8.5% for a leading fashion retailer).
Product Ranking Based on Product Data
As a frequent online shopper myself, I’m constantly relying on the search bar to show me the most relevant results for the item I’m looking for. But if that online retailer is not able to show me the results that are most relevant to what I am looking to purchase, I will leave and go look on another site. If I am searching for ‘ripped straight denim’ and my search results on a retailer’s website show shorts or frayed jeans that are not ripped, when I’d been looking for slashed knees in the jeans, I’ll leave the site and try to find it elsewhere. I’ll bet you will, too.
It all comes down to what is above the fold, and the relevancy and ranking of the product on the search results page. Many search engines will say they offer product ranking based on product data to help drive these more relevant results. However, these results are only going to be as good as the product data that is are feeding it. Most retailers are relying solely on limited, out-of-the box product data, or on manual product attribution that can be time consuming, inaccurate or inconsistent. With Lily AI, we have seen an average 350% increase in attributes per product for the retailers whom we work with. With deeper enriched product data and synonyms built into the taxonomy, a retailer will see immediate impact on the product ranking within search results. The more in-depth and accurate attributes you have for each product, and with proper ranking and filtering, the more relevant your search results will be. So now, thankfully, I can find the ‘ripped straight denim’ I had been looking for.
Context-Supported Search
It’s important for a search engine to be able to contextualize a shopper’s search term. Unfortunately, this is not something most readily provide, and when they do it will be limited in scope. For example, if I search for my beloved ‘trashed denim’, most search engines will not know what I mean by ‘trashed’ – yet if I had searched for ‘ripped denim’ they would have been able to show me results. The search engine would most likely have only been able to show me results if the retailer had called out ‘trashed’ in the actual title or description of the denim. Even semantic search will miss this, as ‘ripped’ and ‘trashed’ are not literal meanings, but customers still use these different keywords to find the same product.
A retailer’s online shoppers who are using the search bar are each very diverse in thought and preference, which makes it important to understand the context of every search and the intent behind it. With Lily AI’s enriched product attribution, which includes domain-specific synonyms, the level and depth of our taxonomy understands that a search for ‘trashed, distressed, destroyed or ripped denim’ can all return similar results. This is because the domain-specific synonyms are able to understand the context of my search.
Trend-Specific Search Terms
With the seasons changing, I am looking to start shopping for my fall wardrobe, and I’m loving the latest trend of ‘study hall’ or ‘dark academia’: think classic button-ups, pleats, argyle and earthy tones. In order for a product to show up on a retailer’s website when I search for items that fall under this trend, the retailer would have needed to identify and attribute products based on the trend. An e-commerce site search engine would not be able to identify products associated with a certain trend unless it is told to do so. This is where Lily AI comes in. Our team of domain experts identifies specific trends, whether seasonal- or micro-based trends, and the retailers who work with us can quickly add the trend to their taxonomy and have their current product catalog tagged with the attributes that match back to the trend. That way, next time I am searching for ‘study hall style sweaters’, the argyle vest and cardigans that are relevant to the trend will show up in my search results.
Hypernym-Hyponyms
In order to bridge the gap between what a shopper types into the search bar and then the challenge of actually connecting them to the right product they want to buy, it is also important for a search engine to have hypernym-hyponyms supported search, so that customer can further refine their search results. If I am doing a general search for ‘sweaters’ and the results returned are over 1,000 items, I would want the ability to further refine my results through filters and facets. A standard e-commerce site search engine will not provide the level of attribute detail to refine your search results down, so this needs to be done by the retailer themselves. Yet there were 1,000 results; you may be asking, how will the retailer know what those filters and facets should be? Lily AI provides the deepest level of attribution that goes beyond parent category alone, and drills further into every product detail allowing a retailer to create filters and facets that will enhance their customers’ shopping experience.
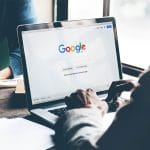